Custom Model Parser
Lumeo is continuously improving the inference support for different model's formats and architectures. However, there might be some cases were Lumeo doesn't support parsing the inference output of some custom or less known architectures. In those cases, you can access the output tensor raw results and parse them in a Function Node.
Below we share the required steps in the model & pipeline configuration, and a snippet of the python code that can be used to access the raw tensors, parse them and store the detected objects and classes in the frame metadata.
Guide
1. Configure the inference model to disable internal parsing
Select Architecture "Other" in the model settings, and "No clustering" as the Clustering algorithm. This disables built-in model output parsers.
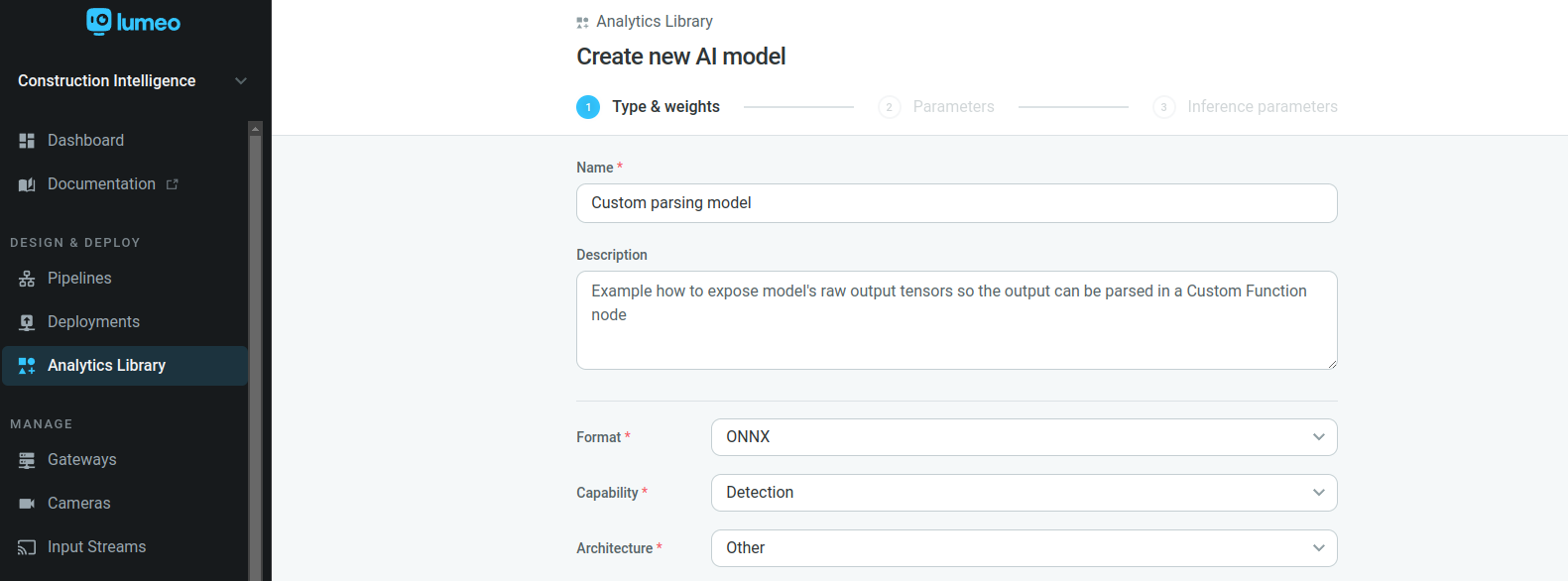
Select "Architecture -> Other" in model "Type & weights" tab
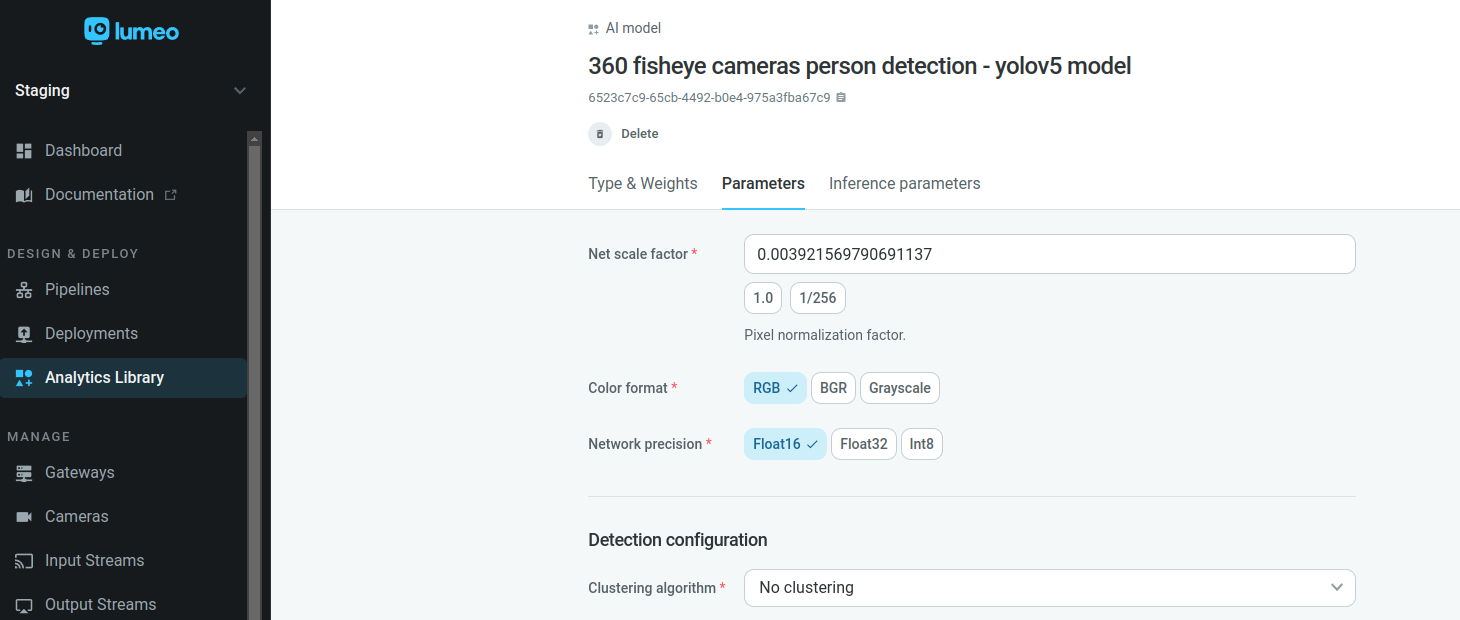
Select "No clustering" on the "Clustering algorithm" of "Parameters" tab
2. Use Custom Function to parse Primary model inference outputs
Primary models are models that operate on the full frame - these models have the "Infer on node" property unset. For such models, add a Custom Function node after the model in question.
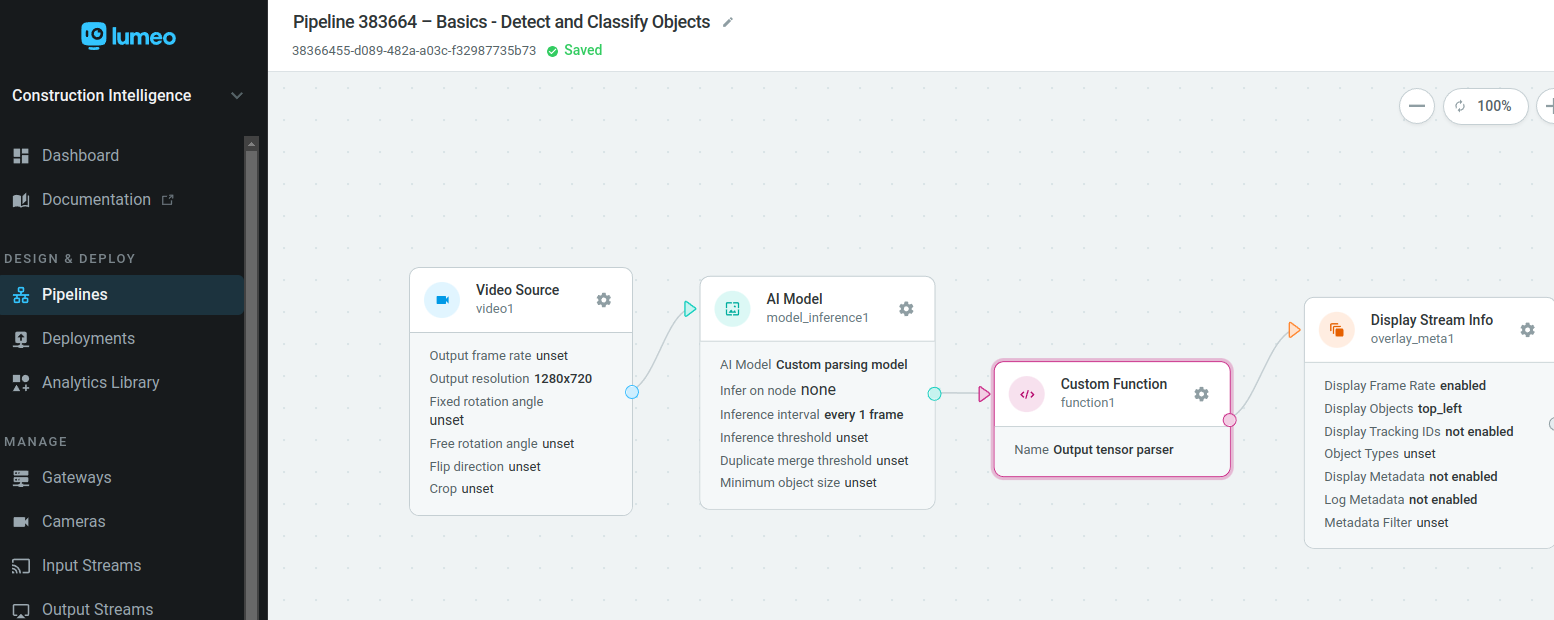
Connect a "Custom Function" after the "AI Model" node
The output tensors from a primary model can be accessed usingframe.tensors()
method within a Custom function. The following code examples show you code for the Custom Function node that parses the output tensors and stores the results in metadata, in a format that can be used by any downstream node.
from lumeopipeline import VideoFrame
import cv2
import numpy as np
# Global variables that persist across frames go here.
# One time initialization code can also live here.
def process_frame(frame: VideoFrame, deployment_id=None, node_id=None, **kwargs) -> bool:
with frame.data() as mat:
# Get the inference model raw output tensor(s)
tensors = frame.tensors()
print("Tensors length = {}".format(len(tensors)))
# Variables where we will store the output tensor as numpy array
heatmaps = pafs = None
for tensor in tensors:
# If there are multiple upstream inference nodes, it might be required filter the tensor by
# the corresponding inference node 'source_node_id'
print("tensor.source_node_id = {}".format(tensor.source_node_id))
for layer in tensor.layers:
print(" Layer name = {}".format(layer.name))
print(" dimensions = {}".format(layer.dimensions))
print(" data = {}".format(layer.data))
# Copy the raw tensor data, filtering by output tensor 'layer.name'
if layer.name == "output_conf:0":
heatmaps = np.asarray(layer.data.copy()).reshape(layer.dimensions)
elif layer.name == "output_paf:0":
pafs = np.asarray(layer.data.copy()).reshape(layer.dimensions)
# Get the height and width of the frame
height, width, _ = mat.shape
if heatmaps is not None and pafs is not None:
###### This step might not be required for your model
# Just an example how to resize the output tensors to half of the size of the input image
heatmaps = np.transpose(heatmaps, (1, 2, 0))
heatmaps = cv2.resize(heatmaps, (int(width/2), int(height/2)), interpolation=cv2.INTER_CUBIC)
pafs = np.transpose(pafs, (1, 2, 0))
pafs = cv2.resize(pafs, (int(width/2), int(height/2)), interpolation=cv2.INTER_CUBIC)
######
current_poses = []
# Insert here the code to parse the output tensors and extract meaningful information (detected objects, classifier results, etc)
#
# Tracking logic and object clustering (for example NMS / Non-maximum Suppression) can also be applied here.
# Save the metadata on Lumeo frame
save_metadata(frame, current_poses)
return True
def save_metadata(frame, current_poses):
try:
# Access frame metadata
meta = frame.meta()
# Get the "objects" field
objects = meta.get_field("objects")
# Iterate over the detected objects on this frame, and create a new object (or update existing ones)
for pose in current_poses:
pose_obj = {
"label": "person",
"class_id": 0,
"probability": pose.confidence,
"rect": {
"left": pose.bbox[0],
"top": pose.bbox[1],
"width": pose.bbox[2],
"height": pose.bbox[3],
},
}
objects.append(pose_obj)
# Save results on Lumeo frame metadata, so it can be access later in downstream nodes
meta.set_field("objects", objects)
meta.save()
except Exception as error:
print(error)
pass
from lumeopipeline import VideoFrame
import cv2
import numpy as np
import json
import torch
import torchvision
# Global variables that persist across frames go here.
# One time initialization code can also live here.
def process_frame(frame: VideoFrame, deployment_id=None, node_id=None, **kwargs) -> bool:
labels = ['animal', 'person', 'vehicle']
with frame.data() as mat:
# Get the inference model raw output tensor(s)
tensors = frame.tensors()
print("Tensors length = {}".format(len(tensors)))
# Variables where we will store the output tensor as numpy array
output_data = xyxy = classes = scores = None
for tensor in tensors:
# If there are multiple upstream inference nodes, it might be required filter the tensor by
# the corresponding inference node 'source_node_id'
print("tensor.source_node_id = {}".format(tensor.source_node_id))
for layer in tensor.layers:
print(" Layer name = {}".format(layer.name))
print(" dimensions = {}".format(layer.dimensions))
#print(" data = {}".format(layer.data))
# Copy the raw tensor data, filtering by output tensor 'layer.name'
if layer.name == "output0":
output_data = np.asarray(layer.data.copy()).reshape(layer.dimensions)
break
# Get the height and width of the frame
height, width, _ = mat.shape
if output_data is not None:
# Insert here the code to parse the output tensors and extract meaningful information (detected objects, classifier results, etc)
detected_objects = []
xyxy, classes, scores = YOLOdetect(output_data) #boxes(x,y,x,y), classes(int), scores(float) [25200]
if xyxy is not None and classes is not None and scores is not None:
# Tracking logic and object clustering (for example NMS / Non-maximum Suppression) can also be applied here.
#nms_output = torchvision.ops.nms(torch.tensor(xyxy, dtype=torch.float),
# torch.tensor(scores, dtype=torch.float),
# 0.5)
# Normalize to input image dimensions 640x640 in this example.
xyxy = xyxy / 640
for i in range(scores.size):
# Filter out objects with low probability
if ((scores[i] > 0.1) and (scores[i] <= 1.0)):
xmin = int(max(1,(xyxy[i][0] * width)))
ymin = int(max(1,(xyxy[i][1] * height)))
xmax = int(min(height,(xyxy[i][2] * width)))
ymax = int(min(width,(xyxy[i][3] * height)))
obj_width = xmax - xmin
obj_height = ymax - ymin
# Filter objects based on min dimensions
if obj_width > 5 and obj_height > 5:
detected_objects.append({
"label": labels[classes[i]],
"class_id": int(classes[i]),
"probability": float(scores[i]),
"rect": {
"left": xmin,
"top": ymin,
"width": obj_width,
"height": obj_height
}
})
#print(json.dumps(detected_objects))
# Save the metadata on Lumeo frame
save_metadata(frame, detected_objects)
return True
def save_metadata(frame, detected_objects):
try:
# Access frame metadata
meta = frame.meta()
# Get the "objects" field
objects = meta.get_field("objects")
# Iterate over the detected objects on this frame, and create a new object (or update existing ones)
for obj in detected_objects:
objects.append(obj)
# Save results on Lumeo frame metadata, so it can be access later in downstream nodes
meta.set_field("objects", objects)
meta.save()
except Exception as error:
print(error)
pass
def classFilter(classdata):
classes = [] # create a list
for i in range(classdata.shape[0]): # loop through all predictions
classes.append(classdata[i].argmax()) # get the best classification location
return classes # return classes (int)
# input is inference_output, output is boxes(xyxy), classes, scores
def YOLOdetect(inference_output):
boxes = np.squeeze(inference_output[..., :4]) # boxes [25200, 4]
scores = np.squeeze( inference_output[..., 4:5]) # confidences [25200, 1]
classes = classFilter(inference_output[..., 5:]) # get classes
# Convert nx4 boxes from [x, y, w, h] to [x1, y1, x2, y2] where xy1=top-left, xy2=bottom-right
x, y, w, h = boxes[..., 0], boxes[..., 1], boxes[..., 2], boxes[..., 3] #xywh
xyxy = [x - w / 2, y - h / 2, x + w / 2, y + h / 2] # xywh to xyxy [4, 25200]
xyxy = np.transpose(np.array(xyxy)) # [25200, 4]
return xyxy, classes, scores # output is boxes(x,y,x,y), classes(int), scores(float) [predictions length]
3. Use a Custom Function to parse Secondary Model inference outputs
Secondary models are models that operate on the objects detected by a primary model - these models have the "Infer on node" property set to the Primary model's node.
Secondary models can be deployed immediately after the Primary model (in which case output is generated for every frame) or subsequent to a Track Objects node after the Primary model (in which case output is generated when the objects change size / position meaningfully). In either case, add a Custom Function node after the model in question to process the secondary model outputs.
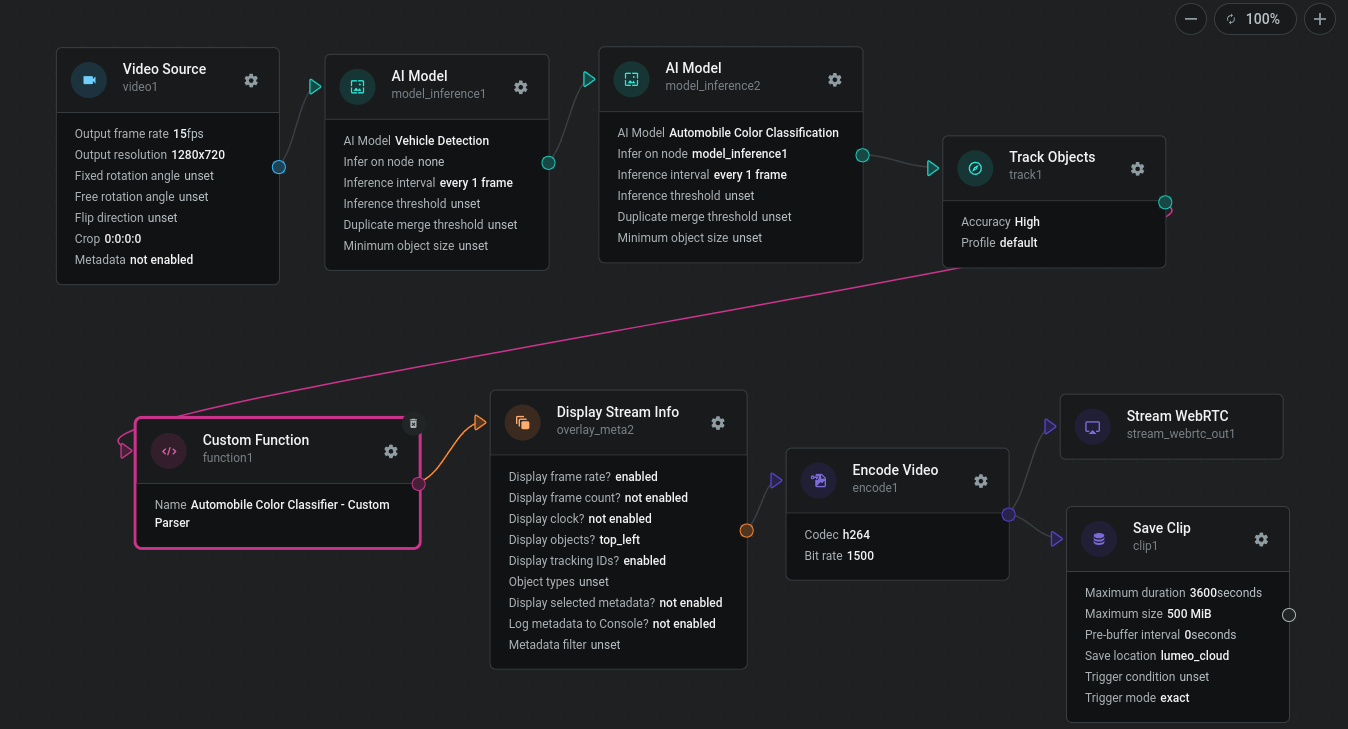
The inference outputs from secondary models are stored inframe.object_tensors()
. The following code snippet shows you how to parse secondary model outputs and save the resulting attributes in the metadata so that it's usable by downstream nodes.
# Note: our inference engine automatically processes the results from Classifiers which output is a softmax layer,
# this code serves just as example to more complex models parsings :)
from lumeopipeline import VideoFrame
import numpy as np
def process_frame(frame: VideoFrame, deployment_id=None, node_id=None, **kwargs) -> bool:
labels = ["black", "blue", "brown", "gold", "green", "grey", "maroon", "orange", "red", "silver", "white", "yellow"]
classifier_min_threshold = 0.5
with frame.data() as mat:
meta = frame.meta()
detected_objects = meta.get_field("objects")
# As the "Color Classifier" inference is performed on top of "Vehicle Detection" model inference results
# the raw output tensor(s) for "Color Classifier" are stored inside Objects tensors
object_tensors = frame.object_tensors()
# Flag to control if we need to store changes to objects metadata
save_objects_metadata = False
if len(detected_objects) > 0 and len(object_tensors) > 0:
for obj, obj_tensor in zip(detected_objects, object_tensors):
if obj["label"] == "car":
for tensor in obj_tensor.tensors:
for layer in tensor.layers:
if layer.name == "predictions/Softmax" and layer.dimensions == [12, 1, 1]:
probabilities = np.array(layer.data).flatten()
max_prob_index = np.argmax(probabilities)
max_prob_value = probabilities[max_prob_index]
if max_prob_value > classifier_min_threshold:
vehicle_color = labels[max_prob_index]
#print(f"Detected {obj['label']} with color {vehicle_color} having probability {max_prob_value:.2f}")
# Add the classifier results a new object's attribute
if "attributes" not in obj:
obj["attributes"] = []
obj["attributes"].append({
"class_id": 25,
"label": f"{vehicle_color}",
"probability": float(max_prob_value)
})
save_objects_metadata = True
if save_objects_metadata:
meta.set_field("objects", detected_objects)
meta.save()
return True
Reference
The following table lists available methods in a Custom Function node to access a model's output layers:
Method | Returns | Description |
---|---|---|
frame.tensors() | A list ofTensor | Access output layers from a Primary model using this method. |
frame.object_tensors() | A list of Tensor | Access output layers from all Secondary models using this method. Secondary tensors can be 1:1 correlated with the objects field in frame metadata to map tensor outputs to object attributes. See example above. |
A Tensor
object has the following properties:
A TensorLayer
object has the following properties
Property | Description | Example |
---|---|---|
name | Name of the model layer, as defined in the Model. | predictions/Softmax |
dimensions | Vector containing the layer's dimensions | [12 1 1] |
data | Flat vector containing the layer's data. You must resize it using the dimensions to obtain a n-d array. | [0.123 0.69 0.009] |
Updated 5 months ago